Treat Injury Prediction like Forecasting the Weather
Much Ado About Injury Prediction
If you live in the sport performance world, much ado has been made over the notion of injury prediction. If only we had a genie in a bottle that could predict an injury, right?
These issues were brought to the forefront recently in a journal article published in Sports Medicine (Hughes, Riley, Callaghan, & Sergeant, 2020). The researchers went back retrospectively to obtain “periodic health examination” data that included measures of lower body joint strength and range of motion from soccer players to see if they could predict muscle injuries.
They found the models to be of poor predictive value and there are several potential reasons for this. But, as we will discuss below, one issue is that many of the continuous variables they measured related to strength and flexibility show overlap between the athletes who suffered injury and those who didn’t.
This weakens the predictive ability of a statistical model.
They also stated that the strength and flexibility predictors tended to vary over time meaning that the measurement’s predictive value would likely decay over time.
The challenges with injury prediction have been discussed in the scientific literature at length (Bahr, 2016; Mørtvedt, Krosshaug, Bahr, & Petushek, 2019).
If We Can’t Predict Injuries, Then What?
Have you ever stopped to think about whether or not injury prediction is what we should be shooting for in the first place and why prediction is so darn hard?
These are fundamentally important questions.
Let’s start with what we really want.
Regardless of the “hat” you wear professionally, an injury prediction model that assigns a probability of injury to an athlete based on a few risk factors doesn’t seem like the complete picture or even an attainable endpoint.
Injuries are highly complex events and distilling this down to what we can measure in a pre-season physical or baseline test seems shrouded with problems.
One thing is clear though — what we should and often do care about is identifying trainable deficits in our athletes. If we know a problem exists, we can then prescribe the right type of individualized training and physical conditioning to bridge the gap. Take an athlete with a reduced left limb explosive strength capacity measured as the rate of force development (RFD). Regardless of how strongly associated or not RFD is to a potential injury subtype (e.g. a knee injury), we often end up doing something about a between-limb RFD deficit because it supports overall athletic performance.
This is the clincher.
We can address trainable physical deficits when we know the problem exists — and in this case, if you aren’t measuring, you’re guessing.
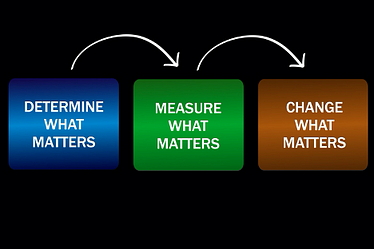
Want to Predict Injuries? Treat them Like the Weather
Now let’s address a couple of reasons why injury prediction is so darn hard.
For starters, injury prediction is tough because the athlete-environment system is a complex adaptive system. Energy is exchanged between the athlete and their environment making it necessary to (a) study the complexity and (b) to get as close as possible to the inciting event as possible to expose potential linkages.
Point (b) is especially important.
I have spent the bulk of the past 10 years of my career trying to improve the neuromuscular testing we do with injured slope sport athletes to help support our decision making around their return to sport readiness.
But did you know that the highest prevalence for an ACL reinjury in alpine skiers is more than 2 years after the first injury?
And guess when athletes are typically tested to determine their readiness for returning to sport?
It’s oftentimes somewhere between 9 and 10 months post-injury!
Our testing timepoints that are there to help us make better decisions around preventing a potential reinjury are typically nowhere near the future injury occurrence itself.
Think about it.
How does a test at 9 months to determine “return to sport readiness” reflect what is actually happening 15 months down the road in time?
It probably has little bearing.
Movement strategies and physiological capacities like RFD and maximal muscle strength change over time. Movement strategies can be considered as emergent physical behaviors that are shaped by the interaction between the athlete-environment complex.
The key is to capture the spontaneous emergence of a strategy or pattern that is problematic and to combine this occurrence with context-specific knowledge to drive decision making. However, in order to do this effectively, we need our monitoring and testing to be somewhat ubiquitous and constant.
Think about the weather.
We get 5 to 10 tornado warnings each summer in Calgary, Alberta — my current city of residence.
But to the best of my knowledge, only a tornado warning in 2000 led to an inciting event that caused significant harm (I admit I didn’t research this point thoroughly but I’m using illustrative language here).
Could you imagine measuring the weather conditions today to make a prediction about the likelihood and potential impact of a tornado that might occur 12 months down the road?
A key characteristic of complex-adaptive systems like the weather or humans moving in their environment is that the emergent behavior is non-linear and shaped by a multitude of factors. A complex-adaptive system cannot be understood by the sum of its parts.
As an aside, this is one reason why simple additive statistical models like multi-variate logistic regressions often fail to assign useful probabilities for the risk of an injury (or reinjury).
How Constraints Shape Our Movement
Just like the probability of a tornado that is shaped by a multitude of factors including the colliding of warm and cold air masses, injury occurrences are also shaped by a myriad of factors. Factors internal to the athlete (i.e. internal constraints), related to the task itself (i.e. task constraints) and factors in the environment (i.e. environmental constraints) shape our movement strategies.
We can think about fatigue as an example of an internal constraint that shapes how we move.
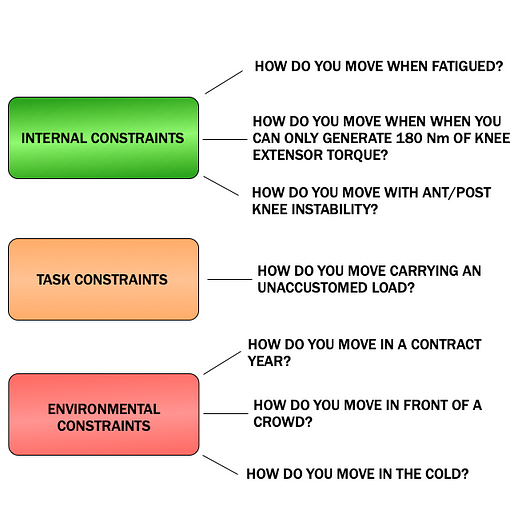
It’s interesting to note that there is only anecdotal evidence to support a link between physical fatigue or performance fatigability (effects of fatigue on performance) and injury. Performance fatigability can be understood as the impact of fatigue processes on the neuromuscular system resulting in a decrease in physical capacities like strength, power and RFD.
But just like the makings of a tornado, combine performance fatigability with low hamstring RFD capacity, a wet field, bad lighting and pressure to win and whammo, you have the makings of a potential injury situation. Even with all of these factors melding together to create the perfect scenario for an injury, the athlete has to be (a) playing the game and (b) exposed to some sort of inciting event (e.g. making an aggressive cut to outplay an opponent) in order to be at risk for an injury. And because we are human and injuries have to do with not only the structural tolerance of the athlete but also a good dose of bad luck, we also find athletes who are strong, rested and fit who will also succumb to injury.
Nothing is certain.
A 60% Chance of Rain! Get My Umbrella
We understand this explicitly when the weather forecaster predicts that there is a 60% chance of rain. If I’m planning my wedding and I’m 14 days out, I will probably start to panic a bit if the long-range forecast predicts a 60% of rain. I would do well here to remember that just like a long-range “injury forecast”, a long-range weather forecast has low accuracy. As we get closer to the wedding day, the uncertainty of the forecast decreases and a lot can change in 14 days.
Even if it says there is a 60% chance of rain one day out from my wedding, I can still hold some hope because a 60% chance of rain means there is a 40% chance it won’t rain.
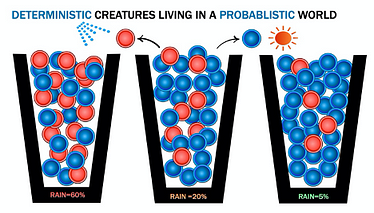
Injury prediction isn’t a genie in the bottle. It’s a probability that gets assigned to a potential outcome that has inherent uncertainty around the estimate.
And what does the weather forecast do for us anyway?
It changes behaviour!
You bring an umbrella to your wedding day or maybe you set up a tent. For the purposes of reducing injuries, when we measure something atypical or find a trend in particular physical capacity or ability that isn’t heading the way we want it to, we change our behaviour and address it, typically through training and exercise.
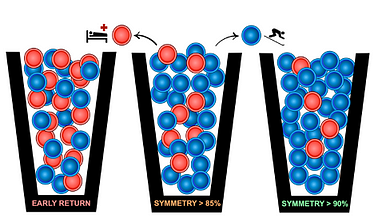
Rather than injury prediction, why not focus on assessments and testing as clue gathering? Think about Sherlock Holmes. He didn’t arrive at the scene of the crime to predict the murder. He arrived there to gather clues so he could arrive at a plausible and likely explanation for the murder.
To this end, pre-season testing, and monitoring enables us to gather clues that allow us to develop more targeted and individualized training programs, and this has a ton of usefulness. You can’t change what you aren’t measuring!
If we start by considering the parallels between injury forecasting and weather forecasting, we see there is a long way to go. Importantly, we need better tools at our disposal to monitor movement patterns in our athletes more ubiquitously.
Wearable Technologies Open New Frontiers to Treat Injuries Like the Weather
This is where Plantiga comes into play.
Plantiga’s approach to movement detection and analysis is a huge milestone because it uses an IMU instrumented insole system and machine learning to make sense of the complexity that comes from the data. Importantly, Plantiga isn’t just reporting acceleration magnitudes or doing rudimentary event detection driven analysis. Instead, the power in Plantiga is the proprietary algorithms that are being developed that extract insights for their users.
With Plantiga we can capture the complexity in the data, acquire measurements all the time including on the field of play where they matter most, and we can do this with ubiquity to drive insights that have previously been impossible to obtain.
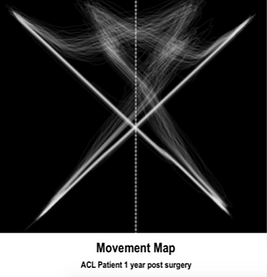
Want to change the world?
Treat the athlete-environment complex and human movement just like how climate scientists treat the weather.
The magic is hidden in the complexity of the data and the variability in what we measure.
Want to develop better insights on what might predict an injury? Then get as close as possible to the event of interest with enough data to study the complexity.
But if you want to get unstuck from the quandary of injury prediction, remember that there is uncertainty in everything we do and that at the end of it all, what we really care about is finding the deficits that athletes are hiding through compensation patterns so that we can design more individualized and targeted training programs.
We may not prevent every single injury, but we will undoubtedly support athlete performance more effectively and we might eventually predict a few injuries along the way.
References
Bahr, R. (2016). Why screening tests to predict injury do not work — and probably never will…: a critical review. British Journal of Sports Medicine J Sports Med, 50(0), 776–780. https://doi.org/10.1136/bjsports-2016-096256
Hughes, T., Riley, R. D., Callaghan, M. J., & Sergeant, J. C. (2020). The Value of Preseason Screening for Injury Prediction: The Development and Internal Validation of a Multivariable Prognostic Model to Predict Indirect Muscle Injury Risk in Elite Football (Soccer) Players. Sports Medicine — Open, 6(1). https://doi.org/10.1186/s40798-020-00249-8
Mørtvedt, A. I., Krosshaug, T., Bahr, R., & Petushek, E. (2019). I spy with my little eye … a knee about to go ‘pop ’? Can coaches and sports medicine professionals predict who is at greater risk of ACL rupture? British Journal of Sports Medicine, 1–6. https://doi.org/10.1136/bjsports-2019-100602